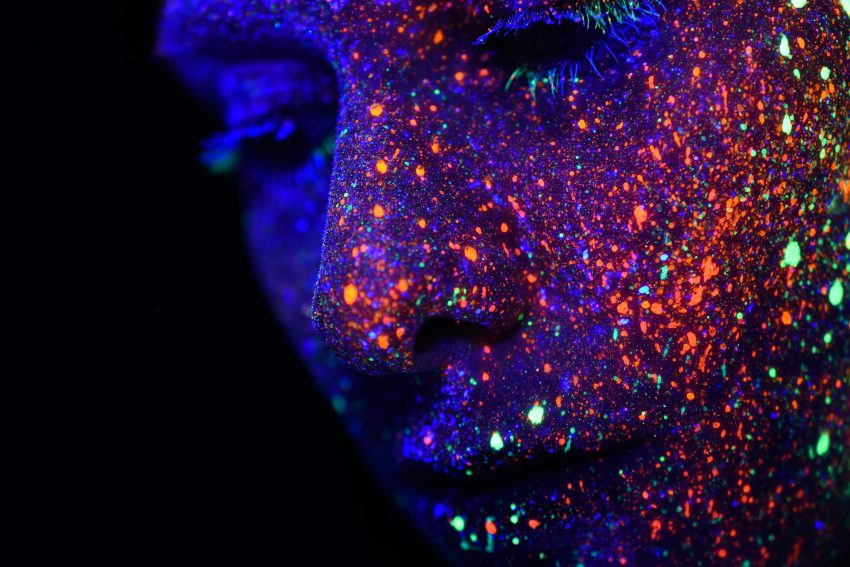
Exploring 7 Machine Learning Models: From Sorting Data to Creative AI
While large language models dominate headlines, various other machine learning approaches thrive. The world of machine learning, with its diverse array of approaches and machine learning models, has become the driving force behind the transformation of raw data into actionable insights. It’s a realm where algorithms are not just tools but intellectual marvels capable of unravelling intricate patterns, understanding human language, and even exhibiting creativity. In this blog, we’ll embark on a comprehensive journey through the landscape of machine learning, unveiling its many facets and probing into the marvels of creative AI.
The Spectrum of Machine Learning
Machine learning, at its core, is a methodology that empowers computers to learn from data. It can be broadly categorised into four major types, each suited to a specific set of challenges:
1. Supervised Learning:
Supervised learning, akin to a seasoned guide, relies on labelled data where each data point is meticulously tagged with known outcomes.
This category excels in predicting outcomes such as loan defaults, sales forecasts, or email categorisation, thanks to its ability to learn from historical data.
2. Unsupervised Learning:
In the uncharted territory of unsupervised learning, algorithms take on the role of explorers, handling unlabelled data to uncover hidden patterns or group similar data points.
Dimensionality reduction, clustering, and outlier detection are among its many applications, making it indispensable for data analysis.
3. Semi-Supervised Learning:
Semi-supervised learning strikes a balance between labelled and unlabelled data, capitalising on the strengths of both worlds.
Predictions on unlabelled data, coupled with high-confidence correctness, are assimilated into the machine learning models, significantly reducing the need for extensive labelling.
4. Reinforcement Learning:
Reinforcement learning, a trailblazer in its own right, trains agents or actors to make optimal decisions in various environments.
This category, distinct from supervised and unsupervised learning, relies on a system of rewards and punishments to teach computers to excel in activities like game-playing and robotic control.
Exploring the Neural Network Revolution
A significant milestone in the evolution of machine learning was the introduction of neural networks, a concept inspired by the intricate architecture of the human brain. These networks, comprising layers of interconnected nodes known as artificial neurons, brought about a revolution in the field. Here’s an in-depth look at this neural odyssey:
1. Neural Networks:
Neural networks, resembling the interconnected neurons in the human brain, use activation functions like sigmoid or ReLU.
Their prowess in supervised learning makes them ideal for tasks ranging from image recognition to natural language processing.
2. Deep Learning:
Deep learning takes neural networks to a whole new level by incorporating an abundance of hidden layers. These layers extract intricate features from the data.
While deep learning opens the doors to a myriad of possibilities, it demands substantial computational resources and often relies on specialised hardware accelerators such as GPUs, TPUs, and FPGAs.
3. Fine-Tuning:
Fine-tuning accelerates model customisation by training only a select few final layers on fresh labelled data. The core weights remain untouched.
Models designed for fine-tuning are aptly termed base models or foundational models, as they serve as the groundwork for specialised applications.
Visionaries in Machine Learning
In the captivating realm of computer vision, deep convolutional neural networks (CNNs) reign supreme. These visionaries possess an extraordinary ability to identify elements within photographs and video frames. They are often trained on expansive photographic datasets, enabling transformative applications across various domains.
The Language of Machines
Language models, a captivating facet of machine learning, harness the power of neural networks to comprehend and generate human language. Their diverse applications encompass translation, grammar analysis, text summarisation, sentiment analysis, and text generation. While they once relied on convolutional neural networks, modern language models predominantly gravitate towards recurrent neural networks (RNNs), long short-term memory (LSTM) networks, and the transformative force of transformers.
Beyond Classification: Unleashing Creative AI
One of the most compelling facets of AI is its ability to transcend mere classification tasks. An AI agent that learns for the sake of learning possesses immense potential, capable of uncovering intricate patterns rather than adhering to predefined tasks. This approach has yielded remarkable outcomes. A notable instance comes from Lawrence Berkeley Lab, where researchers employed the Word2vec text processing algorithm on several million material science abstracts. The algorithm’s mission? To predict discoveries of new thermoelectric materials—a task that extends far beyond binary decisions, ushering in a new era of creative AI.
The Pioneers of Reinforcement Learning
Reinforcement learning, unlike its supervised and unsupervised counterparts, follows a distinctive path. It trains actors or agents to interact with environments in a manner that maximises specific values, often involving a form of trial and error. This intriguing approach finds resonance in training computers to excel in complex games and equipping robots to perform intricate tasks.
The Expansive Landscape of Machine Learning
As we navigate the diverse landscape of machine learning, we encounter a vibrant tapestry of models and methods. From the foundational principles of supervised learning to the transformative capabilities of neural networks and the creative potential of AI, each facet contributes to the evolving narrative of artificial intelligence. It’s a journey marked by continuous innovation, one where machines are not just learning but evolving as creative entities, amplifying human ingenuity in unimaginable ways.
The Future: Where Creativity Meets Machine Learning
The future of machine learning holds boundless possibilities. As AI agents continue to evolve and learn for the sake of learning, we can anticipate astonishing breakthroughs. These breakthroughs will likely transcend our current understanding of what machines can achieve.
In the world of medicine, AI may lead to the discovery of novel treatments for complex diseases, potentially saving countless lives. In the realm of entertainment, AI-driven content generation may produce works of art that resonate with audiences on a profound level. And in the field of scientific research, AI may assist researchers in unravelling the mysteries of the universe, making giant leaps in our understanding of the cosmos.
In conclusion, the landscape of machine learning is an ever-expanding tapestry of innovation and discovery. From its foundational principles to the frontiers of creative AI, this field continues to reshape our world, offering solutions to challenges we once deemed insurmountable. As we journey into the future, we do so with a sense of wonder and anticipation, eager to witness the remarkable ways in which creativity and machine learning will intersect to shape the world of tomorrow.